Product owners and UX researchers often face challenges in understanding interval data. Without clarity, decisions may falter, impacting product development.
Recognizing the significance of precise data comprehension, this guide demystifies interval data. By simplifying concepts, it enables professionals to discern and utilize interval data effectively.
Understanding this data type is pivotal in creating user-centric products and enhancing user experience. This article provides practical insights and examples, facilitating informed decision-making and fostering product innovation.
What is interval data?
Interval data refers to measurements on a scale with equal intervals. It displays both order and direction, aiding in comparisons. However, it lacks a true zero point where zero doesn't signify "none."
This type of data can be added and subtracted, facilitating basic arithmetic operations. Yet, multiplying or dividing it may not yield meaningful results. For product owners and UX researchers, understanding interval data is crucial for making informed decisions.
It allows for structured analysis and interpretation, offering insights into user behaviors and preferences. By grasping its characteristics and limitations, professionals can enhance their strategies and designs effectively.
Now that we have clarified what interval data entails, let's delve into practical examples to grasp its significance in product management.
Examples of interval data
Customer Satisfaction (CSAT) scores and product usage metrics are common examples of interval data in the realm of product management and user experience research. Both types of data provide valuable insights into user preferences, behaviors, and overall satisfaction with the product:
Customer Satisfaction (CSAT) ratings
Customer satisfaction ratings are a common example of interval data in product research. Users are asked to rate their satisfaction level on a scale of 1 to 10, where 1 represents the lowest satisfaction and 10 the highest.
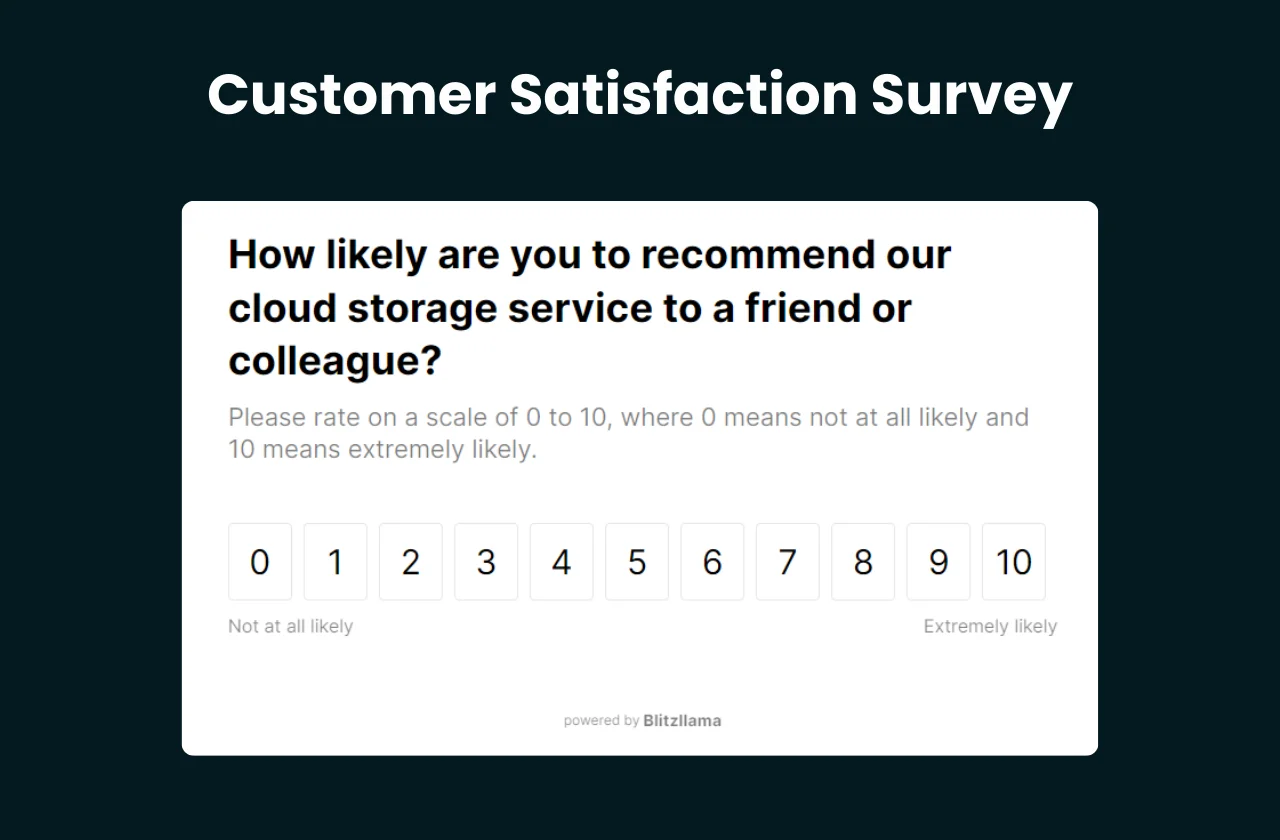
This data provides quantitative insights into how users perceive the product's performance, features, and overall experience. Product owners and UX researchers analyze these ratings to identify areas for improvement and measure the effectiveness of product changes over time.
Understanding the distribution of satisfaction ratings helps in making informed decisions about product enhancements and feature prioritization. Through statistical analysis, trends in customer satisfaction can be identified, allowing teams to proactively address issues and optimize user experience.
Product usage frequency
Another example of interval data is product usage frequency, which measures the number of times users interact with a product within a specified period. This data provides valuable insights into user engagement and behavior patterns. Product owners and UX researchers track usage frequency to gauge the level of user adoption and identify potential usability issues.

Analyzing usage frequency helps in determining the effectiveness of features and identifying areas where users may encounter difficulties or barriers to usage. By monitoring usage patterns over time, product teams can tailor their strategies to enhance user engagement and satisfaction, ultimately driving product success and customer loyalty.
With a clear understanding of interval data examples, let's explore why it holds significant relevance for product owners and managers.
Why interval data matters for product owners and managers?
Interval data serves as a vital tool for product owners and managers to gauge user satisfaction, identify trends, and pinpoint areas for improvement within their products. By analyzing CSAT scores and product usage metrics, stakeholders can make data-driven decisions to enhance user experiences, prioritize feature development, and address pain points effectively:
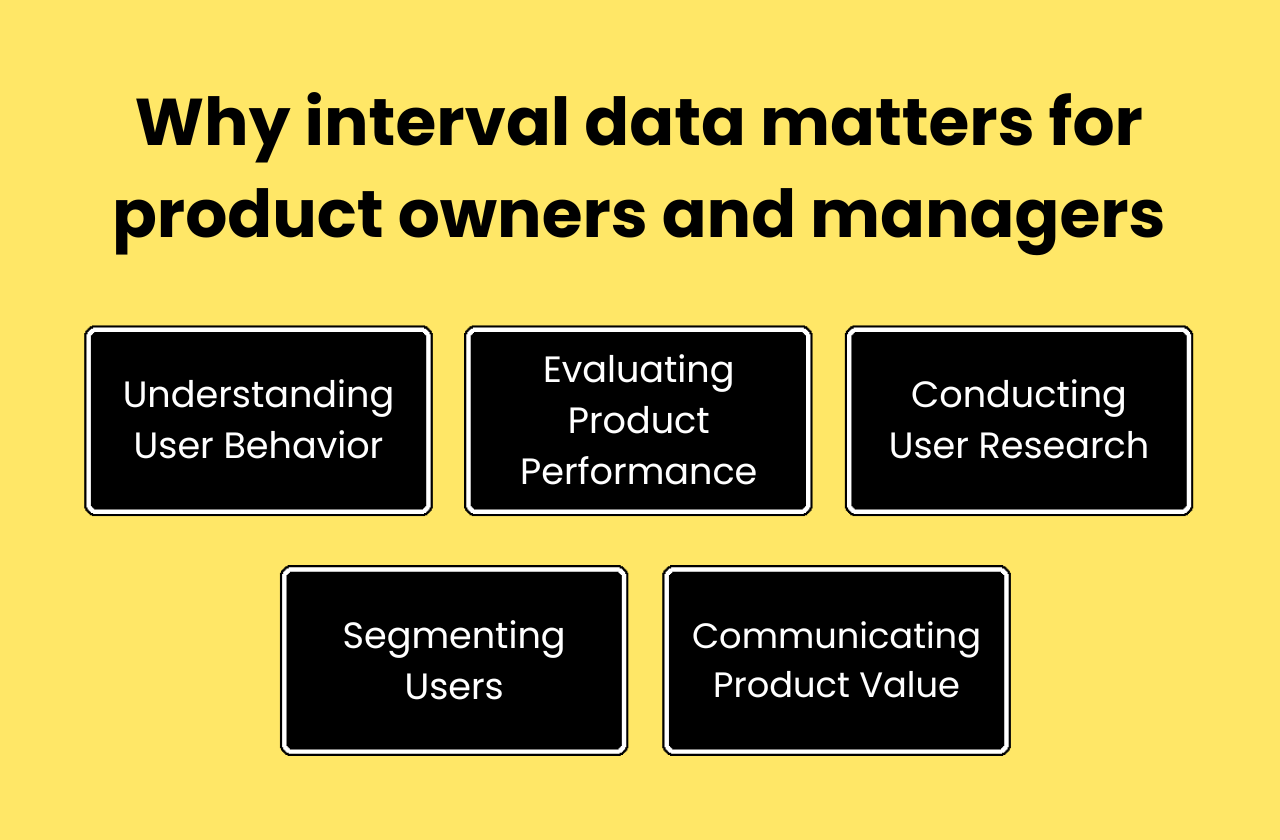
1) Understanding user behavior
Interval data matters for product owners and managers because it helps them analyze user engagement, product usage patterns, and identify areas for improvement.
By tracking user behavior over time, they can see how users interact with the product and pinpoint any bottlenecks or pain points. This insight enables them to make informed decisions about which features to prioritize and how to enhance the overall user experience.
2) Evaluating product performance
Product owners and managers rely on interval data to measure the impact of new features, track key metrics, and make data-driven decisions.
By collecting data at regular intervals, they can monitor how changes to the product affect user behavior and performance metrics. This allows them to iterate quickly, optimize features based on user feedback, and ensure that the product continues to meet the needs of its users.
3) Conducting user research
Interval data is essential for conducting effective user research. Product owners and managers use interval data to design surveys, A/B tests, and user interviews that provide valuable insights into user behavior and preferences.
By collecting data at different points in time, they can track changes in user attitudes and behavior, identify trends, and uncover new opportunities for product improvement.
4) Segmenting users
Interval data enables product owners and managers to segment users based on relevant characteristics such as usage frequency and satisfaction scores.
By grouping users into different segments, they can better understand their needs and preferences and tailor marketing efforts and product development initiatives accordingly. This targeted approach allows them to maximize the impact of their resources and deliver a more personalized experience to their users.
5) Communicating product value
Finally, interval data helps product owners and managers quantify the impact of their product on users and stakeholders. By tracking key metrics over time, they can demonstrate the value of the product in concrete terms and justify investment decisions to stakeholders.
Interval data also enables them to identify areas where the product is performing well and areas where there is room for improvement, allowing them to focus their efforts where they will have the greatest impact.
Now that we recognize the importance of interval data, let's differentiate it from other types of data commonly encountered in product management and research.
Distinguishing interval data from other data types
In product management and UX research, it's essential to distinguish interval data from other data types like nominal, ordinal, and ratio data. Understanding these distinctions enables product owners and managers to interpret data accurately and derive actionable insights:
Comparing interval data to nominal data
Interval data involves measurable quantities with equal intervals, unlike nominal data that categorizes items without any inherent order. In digital products, consider user roles – nominal data defines roles like "admin" or "user" without any order. In contrast, interval data, like user activity levels, measures with consistent intervals, such as "low," "medium," or "high."
Differentiating interval data from ordinal data
While both involve ordered categories, interval data maintains equal intervals, unlike ordinal data where intervals may vary. In software, think of survey ratings – ordinal data captures the order (1st, 2nd, 3rd), while interval data, like Likert scale ratings, assures consistent gaps between responses.
Understanding the relationship between interval data and ratio data
Interval data and ratio data both involve ordered categories, but ratio data introduces a true zero point, enabling multiplication and division. In digital products, consider metrics like user engagement time – interval data might measure the time in minutes, but ratio data allows precise comparisons, like determining one session's duration relative to another.
Now that we've clarified the distinctions, let's explore practical strategies for working with interval data in product management.
Working with interval data in practice
When working with interval data, product owners and managers must employ robust data collection methods, ensure data accuracy and reliability, and utilize appropriate analytical techniques to derive meaningful insights.
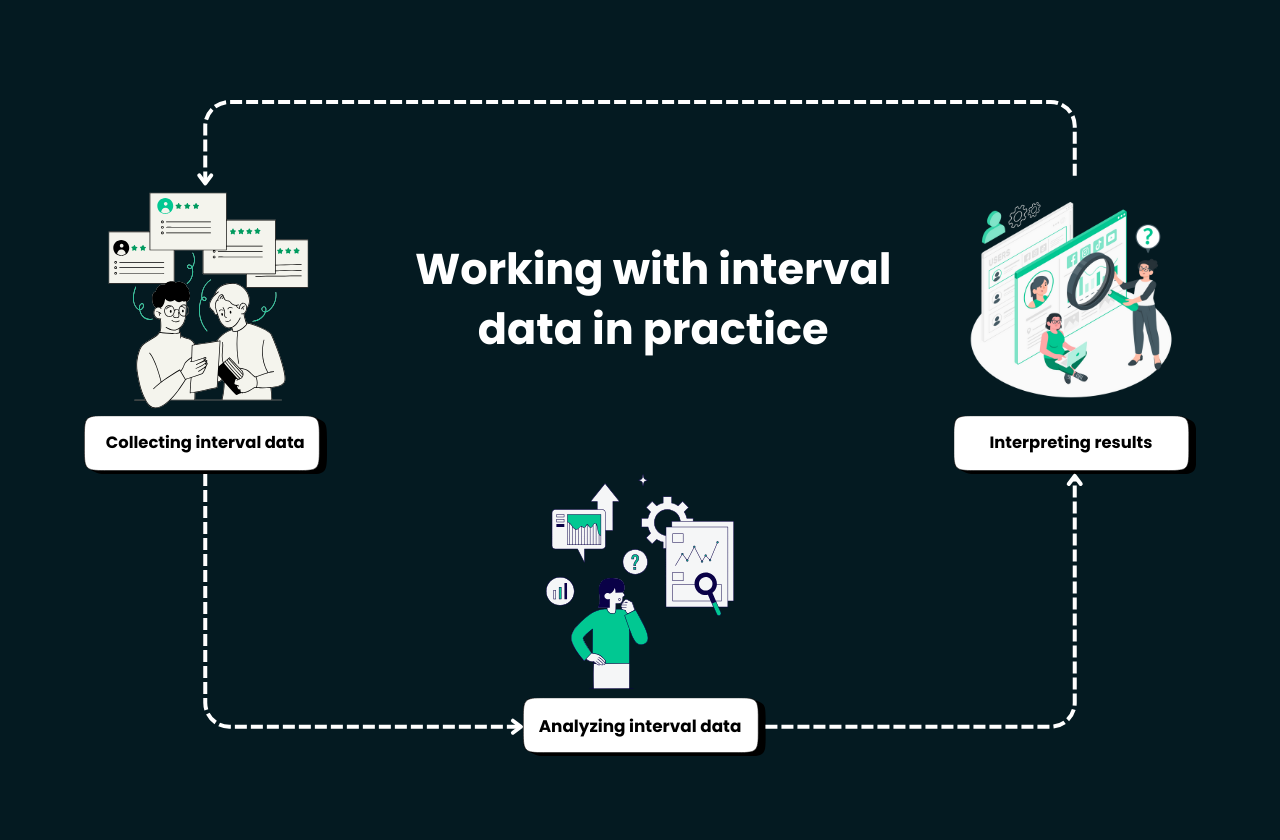
By effectively managing interval data, stakeholders can make informed decisions, optimize product features, and enhance user experiences based on empirical evidence and user feedback:
Step 1: Collecting interval data:
1) Choosing the right techniques.
There are various ways to collect interval data. Some of the most common are:
a. Interviews: Conducting interviews offers flexibility—whether over the phone, face-to-face, or online. Structuring the interview with a standardized set of questions ensures consistency in data collection.
b. Observation: Observation, employing either a naturalistic or standardized approach, involves counting. For instance, tallying the number of attendees at an event during various time frames provides valuable interval data.
c. Surveys: Surveys, conducted through web-based or pen-and-paper questionnaires, are designed to be concise and user-friendly. This method ensures efficient data collection while maintaining ease of navigation.
d. Document reviews: Document reviews prove useful for extracting data from existing documents, be it public records or other historical documents relevant to your research.
e. Probability sampling: Probability sampling involves random selection, enabling researchers to draw probable conclusions based on the collected data.
2) Ensuring data quality and accuracy.
- Rigorously validate the data collection methods to maintain quality and accuracy.
- Implement checks to minimize errors in interview responses or observational counts.
- Verify the authenticity and reliability of documents used in data gathering.
- Regularly assess the randomness and representativeness of the sample in probability sampling.
- Standardize survey questionnaires to enhance clarity and reduce the likelihood of misinterpretation.
Step 2: Analyzing interval data:
Descriptive statistics:
- Utilize mean values to understand the central tendency of the data set.
- Employ median calculations for a robust measure of central tendency, resistant to extreme values.
- Standard deviation provides insights into the variability within the data set, aiding in comprehensive analysis.
Hypothesis testing and statistical significance:
- Apply hypothesis testing to evaluate the validity of assumptions or claims made about the data.
- Establish statistical significance through rigorous analysis, ensuring the observed effects are not mere chance occurrences.
- Utilize p-values and confidence intervals to quantify the degree of certainty in statistical results.
Visualization techniques:
- Create histograms to illustrate the distribution of interval data, providing a visual representation of frequency.
- Boxplots offer a succinct summary of data distribution, highlighting key statistics and potential outliers.
- Employ scatterplots for visualizing relationships and patterns between two variables, aiding in the identification of trends.
Step 3: Interpreting results:
Drawing meaningful conclusions from the data:
- Synthesize findings from descriptive statistics, hypothesis testing, and visualizations to form comprehensive conclusions.
- Relate the results to the initial research questions or objectives, ensuring relevance and applicability.
- Consider the practical implications of the interval data, providing actionable insights for product owners and UX researchers.
Avoiding common pitfalls and misinterpretations:
- Exercise caution in extrapolating findings beyond the scope of the collected data.
- Be aware of potential biases introduced during data collection and analysis.
- Clearly communicate the limitations of the study to prevent misinterpretations.
- Validate conclusions with a cross-disciplinary approach, incorporating feedback from diverse perspectives.
- Continuously refine interpretation methods based on emerging data trends and industry developments.
As we navigate the practical aspects of interval data, let's outline some best practices for utilizing it effectively in product management.
Best practices for utilizing interval data
To effectively utilize interval data, product owners should establish clear objectives, utilize appropriate data analysis techniques, collaborate cross-functionally, and iterate based on continuous feedback and insights derived from the data:
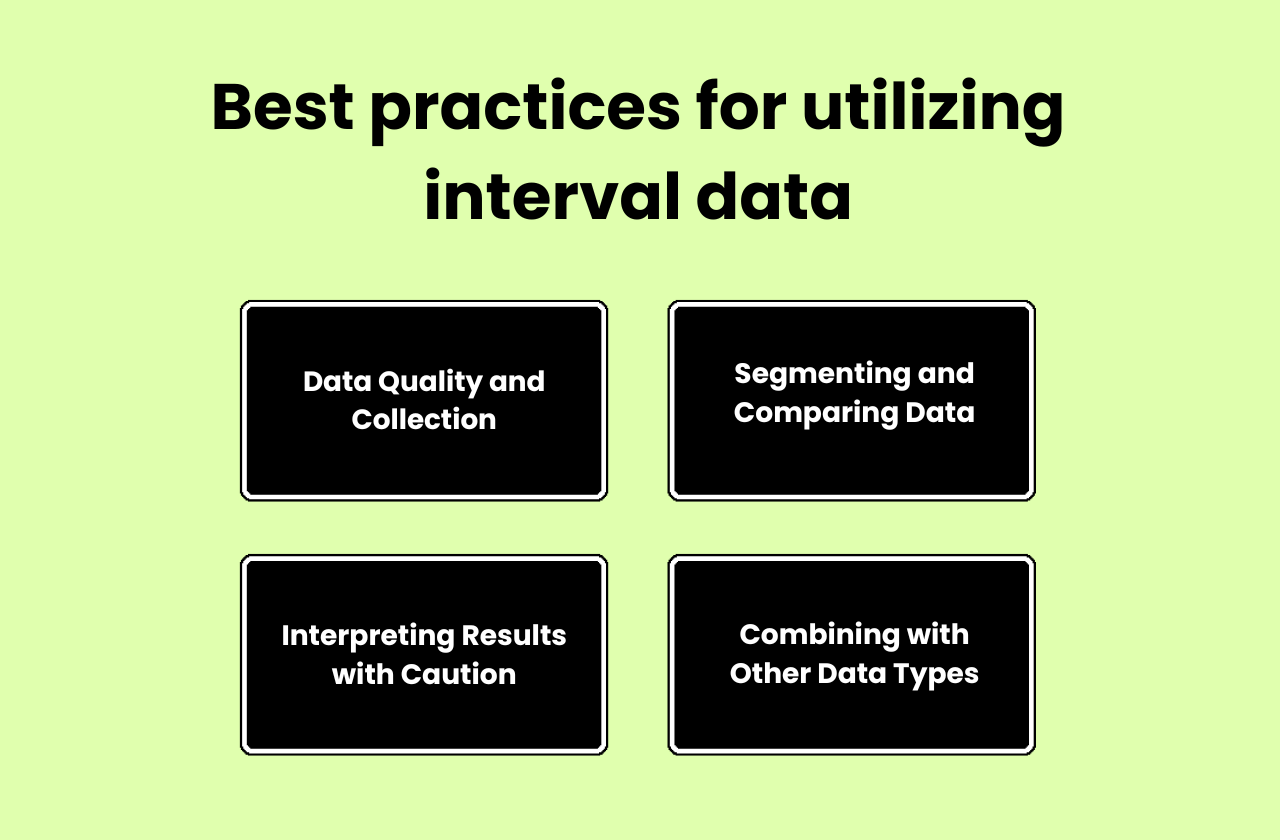
1) Data quality and collection
Accurate data collection methods are crucial. Define clear parameters for gathering interval data. For instance, when tracking user engagement on a website, ensure that the intervals for data collection are consistent.
Use reliable tools and systems to capture data without errors. For example, if monitoring user activity over time, implement timestamp mechanisms to ensure precise interval data collection.
2) Segmenting and comparing data
Segment interval data effectively to derive insights. Divide data into meaningful categories. For instance, when analyzing customer purchase behavior, segment data based on demographics or purchase frequency.
Compare trends across different segments to identify patterns. For example, compare the interval data of new customers versus returning customers to understand their distinct behaviors and preferences.
3) Interpreting results with caution
Acknowledge the limitations of interval data interpretation. Recognize that interval data provides snapshots rather than complete pictures. For instance, when analyzing user engagement metrics, understand that intervals may not capture the full spectrum of user interactions.
Contextual understanding is essential for accurate interpretation. For example, a sudden spike in website traffic may be due to a marketing campaign rather than organic user interest.
4) Combining with other data types
Integrate interval data with qualitative feedback for comprehensive insights. Combine quantitative metrics with qualitative observations. For instance, when evaluating a mobile app's usability, analyze interval data on user interactions alongside user feedback on features and interface design.
This integration provides a holistic view of user experience. For example, if interval data shows high click-through rates but qualitative feedback indicates confusion with navigation, it suggests potential areas for improvement.
Implementing these best practices enables product owners to leverage interval data effectively to enhance product performance and user satisfaction.
Conclusion
In conclusion, interval data offers precise measurements within a scale, facilitating nuanced analysis for product owners and UX researchers. This data type allows for meaningful comparisons and statistical operations, enhancing decision-making processes.
By understanding interval data's characteristics and applications, professionals can leverage its insights to improve product functionalities and user experiences. Its structured nature enables clear interpretations and robust evaluations, contributing to informed design choices.
Moreover, interval data supports the identification of trends and patterns, crucial for refining products and optimizing user interactions. Embracing interval data empowers stakeholders to make data-driven decisions, driving innovation and enhancing user satisfaction.