Introduction
In the fast-paced world of product-driven companies, surveys serve as a critical tool for product and research teams to gather actionable insights and drive meaningful improvements. At tech giants like Airbnb and Stripe, understanding user feedback is a strategic imperative that shapes product development, enhances user experiences, and leads to growth. Through various types of surveys, such as transactional Net Promoter Scores (NPS) after critical product journeys, teams delve into the nuances of user pain points across different stages of the product lifecycle and uncover opportunities to optimize the product.
The power of surveys, especially in-app surveys, lies in their ability to quickly capture highly contextual user feedback and discovering insights. For instance, Airbnb's product team once identified an opportunity to simplify their booking process after a survey revealed that a significant number of users found it overly complex. This insight led to a streamlined booking flow, resulting in improved user satisfaction and increased bookings. Similarly, Stripe leveraged transactional NPS feedback to pinpoint and address specific friction points in the payment process, thereby enhancing their platform's ease of use and reliability for both businesses and their customers.
These examples underscore the transformative potential of well-executed surveys. They are vital instruments that can lead to those "aha" moments, guiding product, user research, and growth teams towards innovations that resonate with users and drive product success. This guide aims to cut through the fluff and provide a direct, comprehensive approach to analyzing survey data, emphasizing the 'how' while understanding the 'why.' By the end of this guide, you'll be turning survey data into a powerful catalyst for product improvement.
Essential Components of Survey Analysis
Step 1 of 5: Ensuring data meets minimal sample size
Why It Matters: Before diving into the analysis, ensuring that your survey data is robust enough to provide reliable insights is crucial. A minimal sample size is necessary to guarantee that the findings are statistically significant and reflective of your broader user base. This step helps avoid skewed interpretations that could lead to misguided decisions.
How to Ensure Adequate Data:
1. Open Text Responses: For qualitative data like open text responses, aim for a diverse set of opinions. While there's no hard rule on the exact number, having responses from at least 1-2% of your target population can provide rich qualitative insight. Tools like thematic analysis can then be used to identify common themes or sentiments.
2. Choices (Multiple Choice, Checkboxes): For questions with predefined options, calculate the sample size needed to achieve a confidence level (typically 95%) and a margin of error (usually 5%). Online sample size calculators can simplify this task by inputting your total user base and desired confidence level. Ensure each choice is represented by a sufficient number of responses to validate trends or preferences.
3. Ratings (Likert Scales, Numerical Ratings): Similar to choice-based questions, use a sample size that allows for a 95% confidence level with a 5% margin of error. This ensures that the average ratings are reflective of the user population's opinions. Additionally, consider segmenting your data to see if different user groups rate experiences differently.
Before the next step of data cleanup:
- Review Distribution: Check the distribution of responses across all questions. This helps identify any biases or concentration of responses that may affect the analysis.
- Segmentation Preparedness: Prepare your data for segmentation by ensuring you've collected relevant demographic or behavioral information. This enables deeper analysis, such as comparing how different user segments perceive your product or service.
Quick example: Number of responses needed for surveys
When running a survey, it's crucial to talk to enough people so that the results reliably represent the larger group's views. Here's a simplified example about how many people you need to survey, assuming a 35% response rate and a 5% drop-off rate between questions:
For 2 question survey: Aim for 602 responses.
For 3 question survey: Aim for 634 responses.
These numbers ensure a 90% confidence level, meaning you can be 90% sure your survey results mirror the broader opinions.
Popular confidence levels for product surveys:
- 90% confidence ;evel: Good for most surveys, strikes a balance between reliability and the number of responses needed.
- 95% confidence level: More common in academic and high-stakes market research, requires more responses but gives higher assurance.
Adjust your survey based on these insights to gather reliable data for informed decision-making.
Step 2 of 5: Data cleanup with specific tactics
Why It Matters: The integrity of your survey analysis depends on the quality of data you analyze. Removing erroneous data such as spam, outliers, and misclicks ensures your conclusions are based on accurate, representative information.
Let’s see below on how to execute data clean-up.
Identifying and removing spam or bot responses:
- Indicator: Completion time significantly below the average (e.g., if the average completion time for a question is 60 seconds, flag responses completed in less than 15 seconds).
- Action: Review these responses manually for nonsensical open-text answers or identical patterns across multiple choice questions, and remove them.
Managing Outliers in Quantitative Data:
- Method: Calculate the Interquartile Range (IQR) for responses to numerical rating questions. The IQR is the difference between the 75th percentile (Q3) and the 25th percentile (Q1) of the data.
- Identification: An outlier is any data point more than 1.5 times the IQR below the first quartile or above the third quartile (i.e., below Q1 - 1.5IQR or above Q3 + 1.5IQR).
- Action: Assess these outliers individually to determine if they represent genuine feedback or if they should be removed due to skewing the data.
Filtering Misclicks and Fast Responses:
- Fast Responses: Establish a minimum threshold based on the 10th percentile of completion times. For example, if the 10th percentile is 15 seconds, ignore responses that are answered less than 15 seconds.
- Misclicks: For NPS or Likert scale questions, a misclick can often be identified when there's a pattern break (e.g., all 5s except a single 1). Manual review of these instances is recommended to determine if they are likely errors.
- Action: For both cases, review the responses in question. If a response seems inconsistent or unengaged, consider excluding it from your analysis.
Performing Consistency Checks Across Related Questions:
- Example: If your survey includes multiple questions about satisfaction in different areas (e.g., service, product quality), but a respondent rates one aspect extremely high and another extremely low, this may warrant a review.
- Action: Use basic statistical analysis to compare responses across these related questions. Significant deviations in patterns without a logical explanation may indicate confusion or misclicks, and these responses may need to be removed.
Practical Tips:
- Automate where possible: Use survey analysis software that can flag potential issues based on the criteria above, saving time in the initial clean-up phase.
- Keep a record: Document the reasons for data removal. This transparency is crucial for validating the analysis process.
Step 3 of 5: Identifying patterns from aggregated data
Why It Matters: Aggregated data analysis allows you to see the forest for the trees, transforming individual responses into a coherent overview of your user base's opinions, preferences, and experiences. This step is pivotal in identifying general trends, common themes, and overall satisfaction levels.
How to Extract Patterns:
- Aggregate Responses: Use statistical measures like mean, median, and mode to summarize responses to quantitative questions. For example, average ratings for a feature can highlight its perceived value or areas needing improvement.
- Visualize Data: Employ visual tools like bar charts for choice-based questions or histograms for rating scales to easily spot trends and distributions. Visualization makes it easier to communicate findings to stakeholders.
- Segment Analysis: Break down data by user demographics or behavior segments. This could reveal how different groups experience your product differently, leading to more targeted improvements.
Step 4 of 5: Conducting cross-question analysis
Why It Matters: Cross-question analysis helps uncover correlations between different aspects of the responses. For example, 85% of users who choose Option A in Question 1, rate 8 and above on NPS.
How to Conduct Cross-Question Analysis:
- Correlation Analysis: Use basic statistical techniques to explore relationships between different survey questions. For instance, see if users who rate the onboarding process highly also report higher overall satisfaction.
- Pattern Recognition: Look for patterns in open-ended responses related to specific ratings or choices. This can help explain the "why" behind quantitative scores.
- Hypothesis Testing: Formulate hypotheses based on your initial analysis (e.g., "Users who find the payment process easy are more likely to recommend our service") and use cross-tabulation to test these theories.
Step 5 of 5: Merging survey responses with quantitative data
Why It Matters: This step enhances strategic decision-making by integrating user feedback with data analysis to identify key insights and trends. It helps pinpoint which user groups are most or least satisfied and how perceptions vary across demographics, informing targeted product development and marketing strategies.
How to execute the analysis:
- Segmentation: Organize survey responses by user attributes like location, usage frequency, or customer value. This sets the foundation for detailed analysis.
- Data Merging: (i) Quantitative Analysis: Analyze numeric data to identify trends and averages within each segment. (ii) Qualitative Analysis: Use text analysis to categorize open-ended responses by theme or sentiment. (iii) Combining Insights: Integrate quantitative and qualitative findings to gain a full understanding of user feedback.
- Identifying Patterns: Employ statistical tools to discover correlations or causal links, such as whether high-value customers report higher satisfaction.
- Actionable Insights: Translate findings into actionable strategies, like improving engagement for certain user groups or addressing specific feedback from a demographic.
Practical Applications:
- Visualization: Use charts and graphs to make data-driven insights accessible.
- Feedback Integration: Share findings across teams to refine product and marketing strategies continuously.
By effectively merging survey data with quantitative and qualitative analysis, teams can derive actionable insights to drive product innovation and enhance user satisfaction.
Automate your survey analysis with Blitzllama
Blitzllama automates survey analysis, transforming complex data into actionable insights effortlessly. Here’s how:
- Data Clean-Up: Blitzllama’s advanced module automatically filters out responses with outlier completion times and identifies spam, ensuring the data you analyze is both accurate and relevant.
- Automatic Tagging: Say goodbye to manual categorization. Blitzllama tags open text responses into topics automatically, saving you countless hours of analysis.
- Product Taxonomy Alignment: These topics aren't just generic; they're aligned with your product's specific taxonomy, making the insights you gain directly applicable to your strategy.

- Survey Level Insights: Beyond simple analytics, Blitzllama performs cross-question analysis at the survey level, uncovering complex insights and patterns without manual intervention.
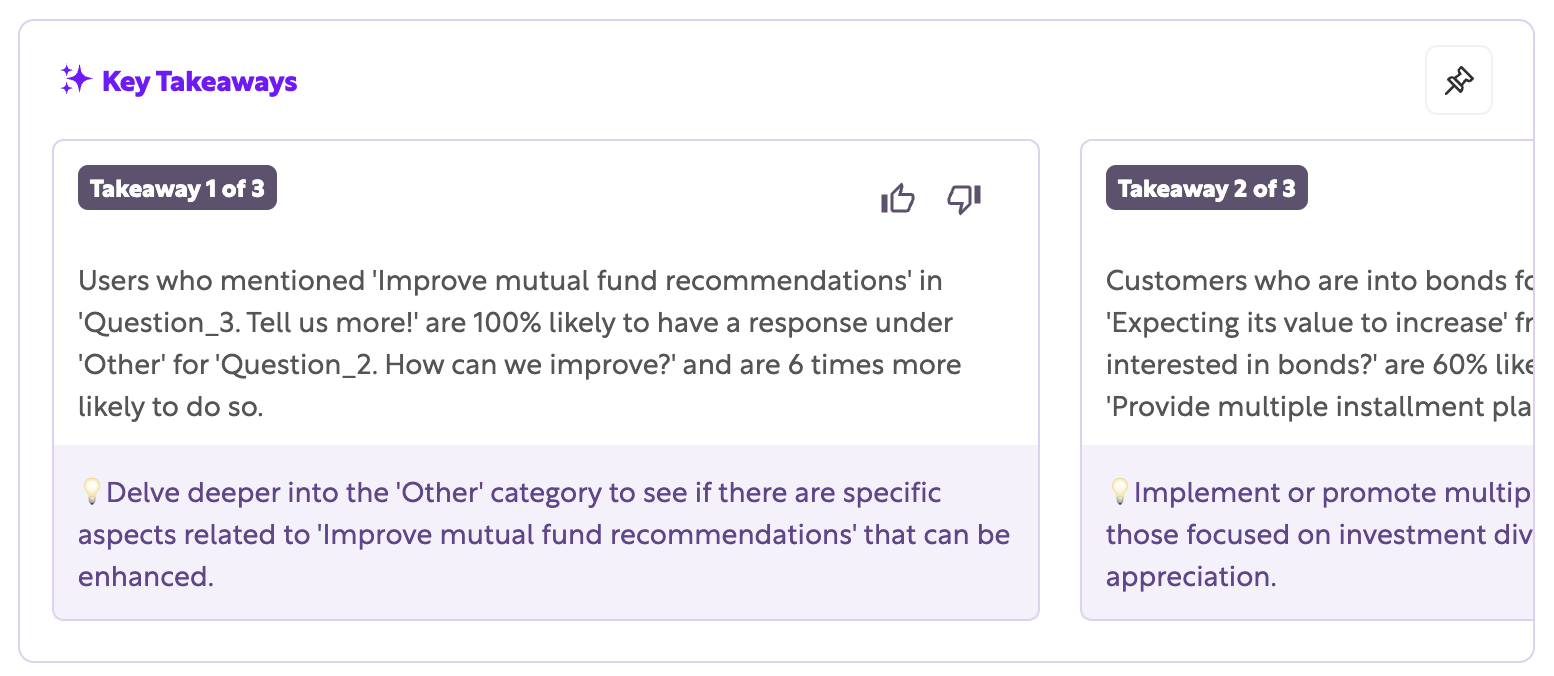
- Shareable Reports: Generate concise, insightful reports with the click of a button. These reports are shareable via links, making collaboration and presentation straightforward and efficient.
With Blitzllama, diving deep into user feedback and emerging with strategic insights has never been easier or faster. Sign up for free and start transforming your survey data into actionable steps for your product's success. Explore Blitzllama now and bring precision and depth to your product analysis.