Many surveys face a common challenge: Bias.
Identifying the most vulnerable surveys is crucial for product owners and UX researchers striving for accurate insights.
Bias can infiltrate subtly, distorting results and leading decision-makers astray.
Imagine putting in the effort to gather data only to realize it's skewed.
This article sheds light on identifying the surveys most susceptible to bias.
Understanding these pitfalls is the first step toward unbiased data collection.
We offer practical insights to help product owners and UX researchers navigate the maze of bias, ensuring their surveys deliver reliable and meaningful results.
What is survey bias?
Survey bias occurs when the way questions are framed or presented influences participants' responses, skewing data accuracy.
This bias can stem from leading questions that prompt specific answers or wording that unintentionally sways opinions.
Product owners and UX researchers must be vigilant, as biased surveys can misguide decisions. Detecting and mitigating bias ensures reliable insights for informed product development.

Crafting neutral, clear questions, and avoiding leading language are essential practices to minimize survey bias, ensuring data reflects genuine user perspectives.
Now that we grasp the concept of survey bias, let's explore how its subtle influence can significantly alter the course of research and shape the outcomes.
How can survey bias influence research and results?
Survey bias exerts a substantial impact on research outcomes, influencing the conclusions drawn from collected data. Whether through leading questions, respondent selection, or other factors, these biases can subtly distort the reality researchers seek to understand:
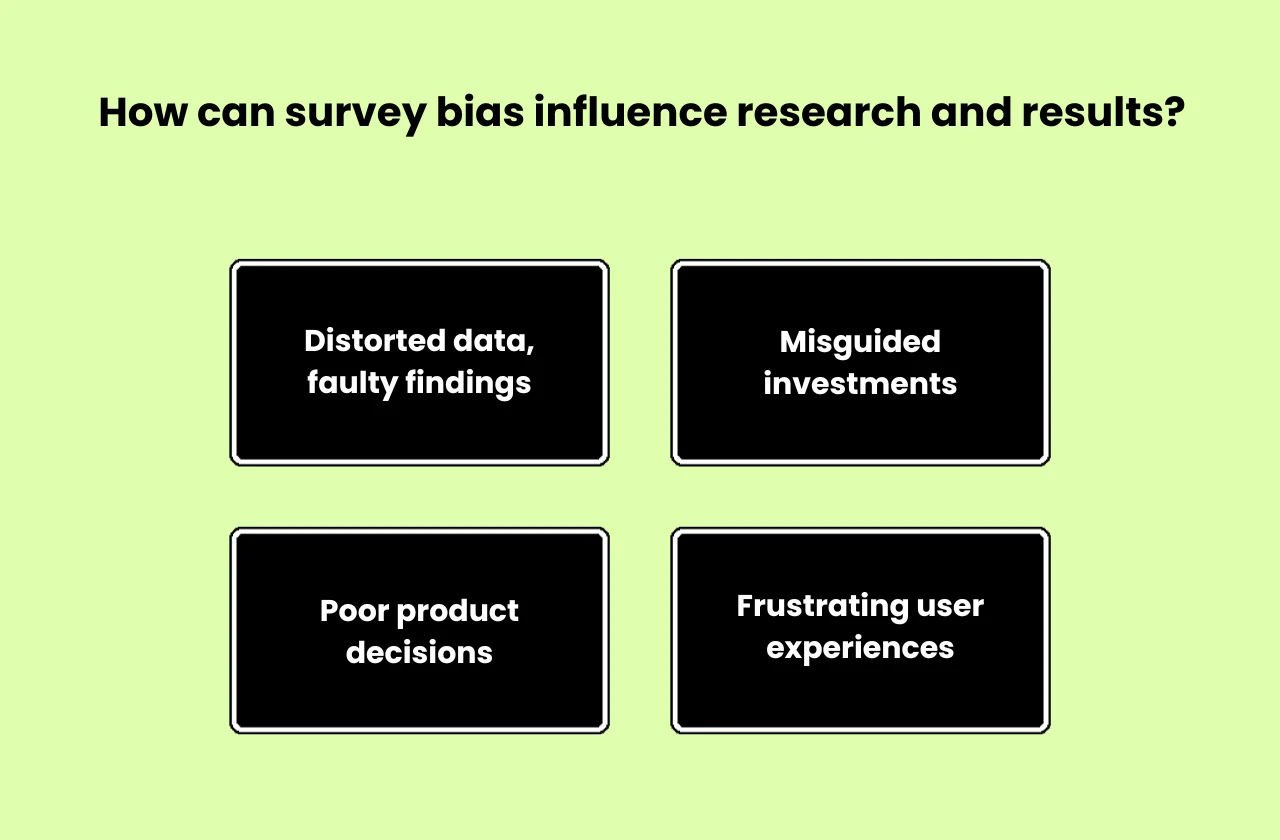
1) Distorted data, faulty findings
Survey bias can significantly impact the quality of collected data, leading to distorted findings.
When surveys are designed with unintentional biases, respondents may feel nudged toward certain responses or may be excluded altogether.
This distortion compromises the accuracy of the data, resulting in faulty findings.
For product owners and UX researchers, this means basing decisions on unreliable information, potentially leading to misguided product development strategies.
2) Poor product decisions
Survey bias can have a direct and adverse effect on the decision-making process, particularly in product development.
When biased data is used to inform product decisions, it can lead to poor choices and the development of features that may not align with the actual needs and preferences of users.
This misalignment can result in the creation of products that fail to resonate with the target audience, leading to decreased user satisfaction and, ultimately, impacting the product's success in the market.
3) Misguided investments
The influence of survey bias extends beyond product decisions to impact resource allocation and investments.
If research is based on biased data, organizations may make misguided investments in areas that do not align with the genuine needs of their target audience.
This misallocation of resources can lead to financial losses and hinder the overall growth and success of the business.
For product owners and UX researchers, ensuring unbiased survey design is crucial to making informed and strategic investment decisions.
4) Frustrating user experiences
Survey bias can also seep into the user experience (UX) domain, affecting how products are perceived and used.
Biased data can result in the development of features that may frustrate users rather than enhance their experience.
When products are designed based on skewed information, they may not cater to the actual preferences and behaviors of the users, leading to dissatisfaction and frustration.
For product owners and UX researchers, addressing survey bias is essential to creating products that genuinely meet user expectations and provide a positive and seamless experience.
To gain a deeper understanding of survey bias, let's explore the different types that researchers encounter in their quest for accurate data.
Types of survey bias
Survey bias manifests in various forms, each presenting unique challenges to researchers. From sampling bias to non-response bias, recognizing these types is the first step towards minimizing their impact on research findings.

Delving into the intricacies of each bias type equips product owners and UX researchers with the knowledge needed to navigate the complexities of data collection:
1) Sampling bias:
Sampling bias occurs when the sample chosen for a survey is not representative of the entire population. This can lead to skewed results, as the opinions or characteristics of the selected group may not accurately reflect those of the broader audience.
Example:
Imagine a tech company conducting a user satisfaction survey but only distributing it to customers who recently purchased their flagship product. If the survey excludes long-term users or those who encountered issues, the results may not truly represent the diverse experiences of all customers.
2) Recall bias:
Recall bias arises when survey respondents provide inaccurate or incomplete information due to memory limitations. People may forget certain details or events, leading to a distorted representation of their experiences.
Example:
Consider a travel app conducting a survey about users' recent vacations. If respondents are asked to recall specific details about their trip, such as expenses or places visited, their responses may be influenced by memory lapses, resulting in an incomplete or inaccurate portrayal of their travel experience.
3) Recency bias:
Recency bias occurs when respondents disproportionately emphasize recent events or experiences in their responses, overshadowing more distant memories. This bias can lead to an overemphasis on the most recent occurrences rather than a balanced assessment of the overall experience.
Example:
In a streaming service satisfaction survey, if users are prompted to rate the platform based on their most recent interaction, they may overlook previous positive experiences or persistent issues. This bias can skew the survey towards a limited timeframe rather than capturing the user's overall satisfaction.
4) Social-desirability bias:
Social-desirability bias occurs when respondents provide answers that they believe are socially acceptable or expected rather than expressing their true opinions. This bias often stems from a desire to conform to societal norms or avoid judgment.
Example:
Consider a fitness app asking users about their exercise habits. Respondents may be inclined to exaggerate the frequency and intensity of their workouts to present a more favorable image, leading to an inaccurate representation of their actual fitness behaviors.
5) Prestige bias:
Prestige bias occurs when respondents provide answers that align with perceived societal or professional norms, potentially overstating their preferences or behaviors to appear more sophisticated or knowledgeable.
Example:
In a survey about reading habits, respondents may indicate a preference for classic literature or acclaimed authors over popular genres. This could be influenced by a desire to project a certain level of cultural sophistication, leading to an overrepresentation of high-prestige preferences in the survey results.
6) Acquiescence bias:
Acquiescence bias occurs when respondents consistently agree with survey statements without carefully considering or evaluating each item. This bias can arise from a tendency to agree with statements or a desire to please the surveyor.
Example:
In a customer feedback survey, if respondents frequently choose the "agree" option without carefully considering each statement, it may indicate acquiescence bias. This can result in a lack of meaningful insights, as respondents may not differentiate between statements that genuinely reflect their experiences and those that do not.
7) Question order bias
Question order bias occurs when the placement of a question influences how respondents answer it, leading to a skewed representation of their true opinions or experiences. The order of questions can shape respondents' thoughts, affecting their subsequent responses.
Example:
In a survey for a project management app, placing a question about overall satisfaction at the beginning might prompt users to focus more on positive aspects, even if they had specific complaints about certain features. On the other hand, placing the satisfaction question at the end might lead users to remember negative experiences more vividly.
8) Current-mood or Emotional-state bias
Current-mood or emotional-state bias arises when respondents' emotions at the time of taking the survey significantly impact their responses. Emotional states can influence the perception of experiences and affect the feedback given.
Example:
Consider a customer feedback survey for a meditation app. If users are prompted to rate the app's effectiveness immediately after a successful meditation session, they might overemphasize positive aspects due to their elevated mood. Conversely, if the prompt follows a frustrating experience, it could lead to a more critical evaluation.
9) Central-tendency bias
Central-tendency bias occurs when respondents consistently choose responses near the middle of the scale, avoiding extreme options. This can happen when users are hesitant to express strong opinions or when the survey design lacks clarity.
Example:
In a satisfaction survey for an e-commerce platform, users might habitually select the middle rating, even if they have strong feelings about the service. This could obscure genuine concerns or positive feedback, making it challenging to identify areas that need improvement.
10) Random-response bias
Random-response bias occurs when respondents answer survey questions haphazardly, without thoughtful consideration. This type of bias undermines the reliability of the data collected, as responses may not accurately reflect users' true opinions.
Example:
Imagine a survey for a weather app where users are asked about specific features, and some users randomly select options without paying attention. This random response can lead to misleading insights into which features are genuinely valued or disliked by the user base.
11) Wording bias
Wording bias stems from the way questions are framed, leading respondents to interpret them in a specific manner. It can unintentionally guide users towards certain responses, potentially distorting the true range of opinions.
Example:
In a survey for a finance app, asking, "How easy is it to manage your expenses with our intuitive interface?" may result in more positive responses compared to rephrasing the question as, "Do you find our interface easy to use for managing expenses?" Wording can subtly influence perceptions and bias the feedback received.
12) Non-response bias
Non-response bias occurs when certain groups of users are less likely to participate in a survey, leading to an unrepresentative sample. Users who choose not to respond might have different opinions or experiences than those who do, introducing bias into the collected data.
Example:
In a survey for a productivity app sent via email, if users who are dissatisfied with the app are less likely to open and complete the survey, the feedback received might disproportionately represent the views of satisfied users. This non-response bias can skew the overall understanding of user satisfaction levels.
Now that we've identified the types of survey bias, let's pivot our focus to the different survey methodologies and their susceptibility to bias.
What are the types of surveys?
Understanding the various survey methodologies is essential for researchers to choose the most appropriate approach for their study.
Different types of surveys, such as in-product surveys, telephone surveys, or anonymous surveys each come with their own set of challenges regarding survey bias.
Unraveling the nuances of survey types is crucial for devising effective strategies to minimize bias:
1) In-product surveys
In-product surveys are a direct way to gather user feedback within a software or application interface.
Product owners can integrate these surveys seamlessly into their platforms, making it convenient for users to share their thoughts without leaving the application.
For example, tools like Blitzllama and Pendo enable product teams to design and deploy in-product surveys, asking users questions about their experience, preferences, or specific features.
These surveys are effective in capturing real-time insights as users interact with the product.
Product owners can inquire about usability, identify pain points, or gauge user satisfaction.
In-app surveys are particularly valuable for understanding the user experience at different touchpoints and making informed decisions to enhance the product.
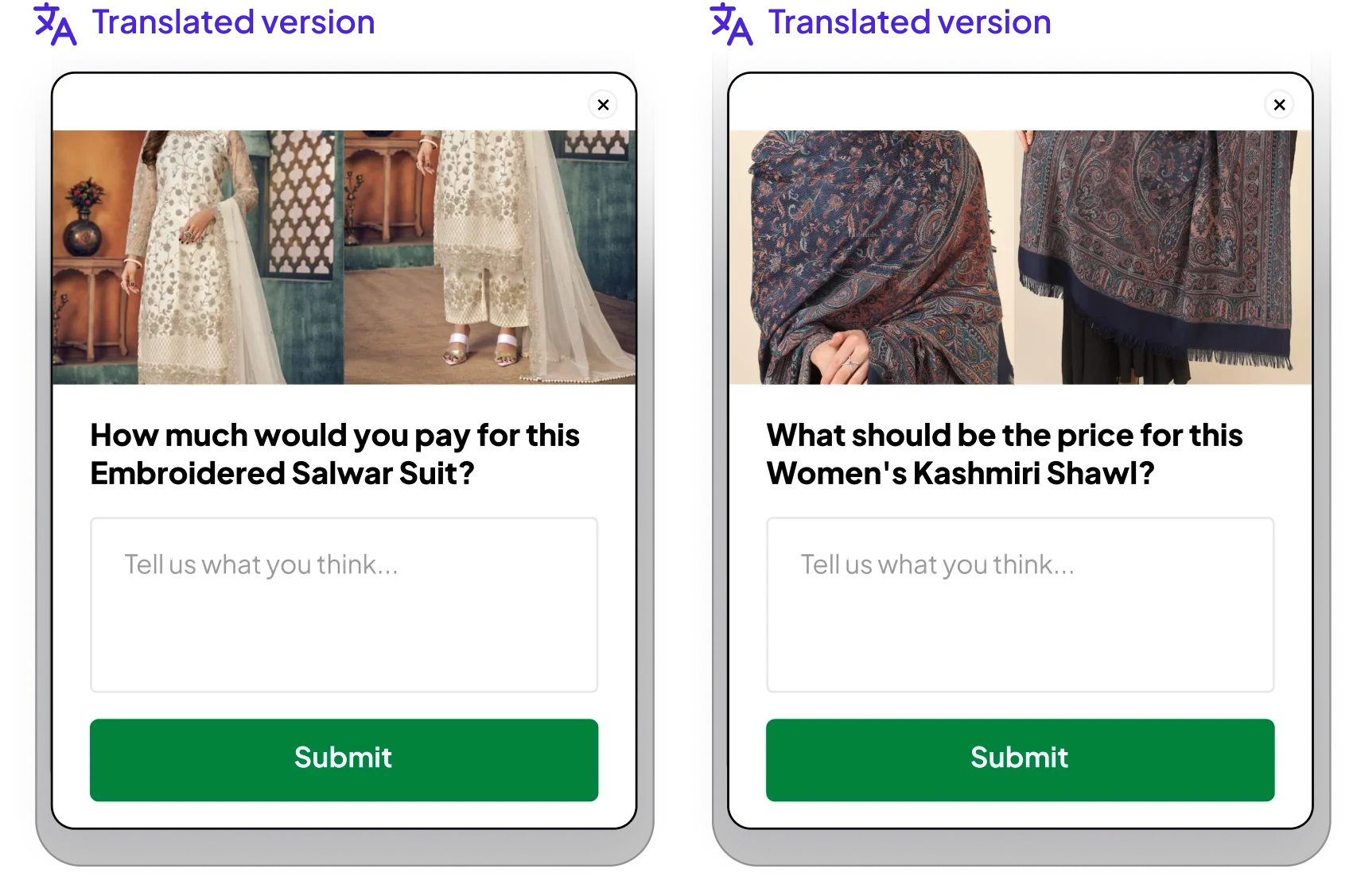
2) Email surveys
Email surveys involve sending targeted questionnaires to users via email.
This method allows product owners to reach a broader audience, including those who may not be actively using the product at a given moment.
SurveyMonkey and Typeform are examples of tools that facilitate the creation and distribution of email surveys.
Through email surveys, product owners can gather feedback on overall satisfaction, feature preferences, or reasons for disengagement.
The structured format of email surveys makes it easy for users to respond at their convenience.
This approach is useful for obtaining insights from a diverse user base and understanding how the product aligns with different user needs and expectations.

3) Anonymous online surveys
Anonymous online surveys provide a platform for users to share their opinions without revealing their identities.
This type of survey encourages candid responses, as users may feel more comfortable expressing their thoughts without the fear of repercussions.
Google Forms and SurveyMonkey offer options to create anonymous surveys, ensuring a safe space for honest feedback.
Product owners can leverage anonymous surveys to gather insights on sensitive topics, assess overall user sentiment, or understand the impact of specific features.
By preserving user anonymity, this survey type fosters a more open and transparent channel for users to communicate their perspectives, leading to valuable data for product improvement.
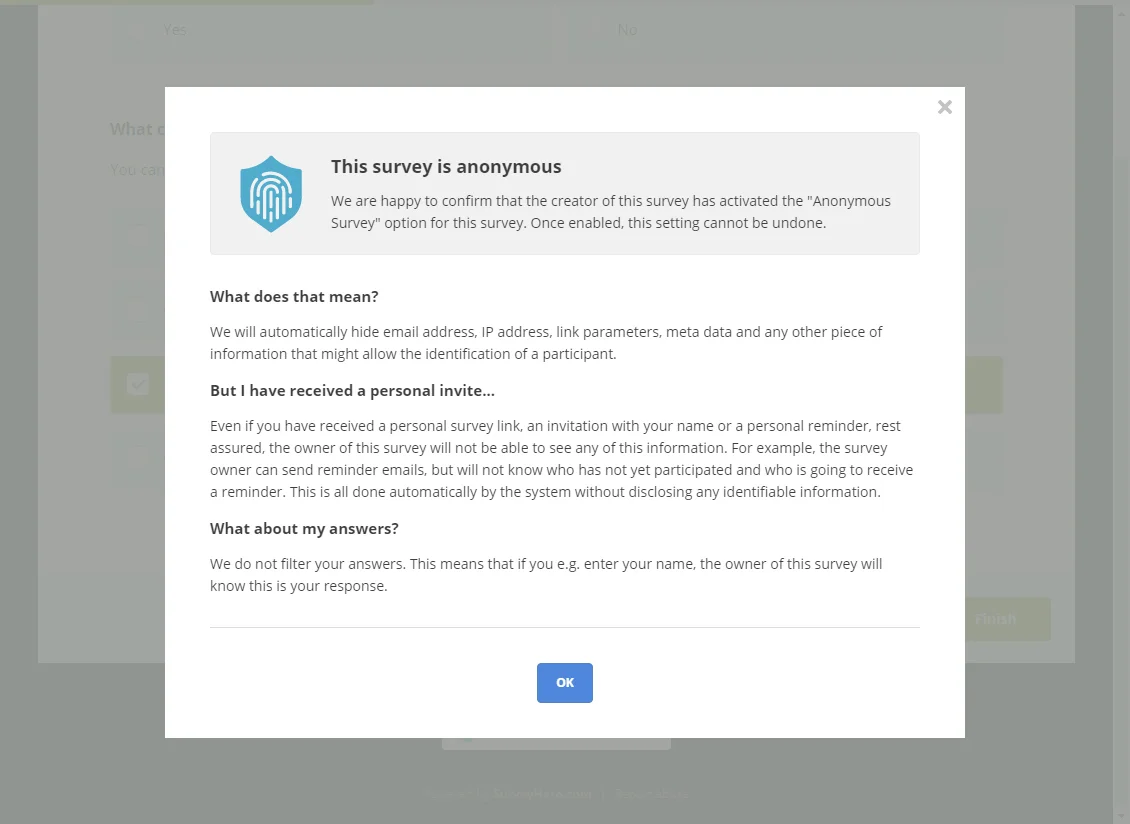
4) Link surveys (Distributed over messaging tools or forums)
Link surveys involve sharing survey links through messaging tools, forums, or social media platforms.
This method allows product owners to reach users where they are most active.
Tools like Blitzllama and SurveyGizmo enable the creation of surveys with shareable links for distribution.
By leveraging messaging apps or forums, product owners can tap into the community aspect of their user base.
This approach is effective for collecting feedback from specific user segments or communities, such as beta testers or power users.
Link surveys facilitate a broader reach and can be strategically distributed to gather insights from different user groups.

5) Surveys over phone calls
Surveys conducted over phone calls provide a personal touch to gathering user feedback.
While this method may require more resources, it offers a direct and interactive way to understand user perspectives.
Tools like SurveyMonkey and CallHub support the creation and execution of phone surveys.
Phone surveys are particularly beneficial for diving deep into user experiences, addressing specific concerns, and clarifying ambiguous feedback.
This approach allows product owners to establish a direct connection with users, gathering qualitative insights that might be challenging to capture through other survey methods.
Phone surveys are especially valuable when aiming for a more in-depth understanding of user sentiments and experiences.

As we explore survey types, it's imperative to pinpoint which surveys are most likely affected by bias, what type of bias, and strategies to avoid its influence. Let's delve into this crucial aspect of survey research.
Which survey is most likely affected by bias (what type of bias and how to avoid it)?
Certain survey types are more susceptible to specific biases. Identifying these vulnerable areas and implementing strategies to counteract bias are vital for accurate data collection.
In this section, we dissect which surveys are most prone to bias, the nature of that bias, and practical approaches to steer clear of its distorting effects:
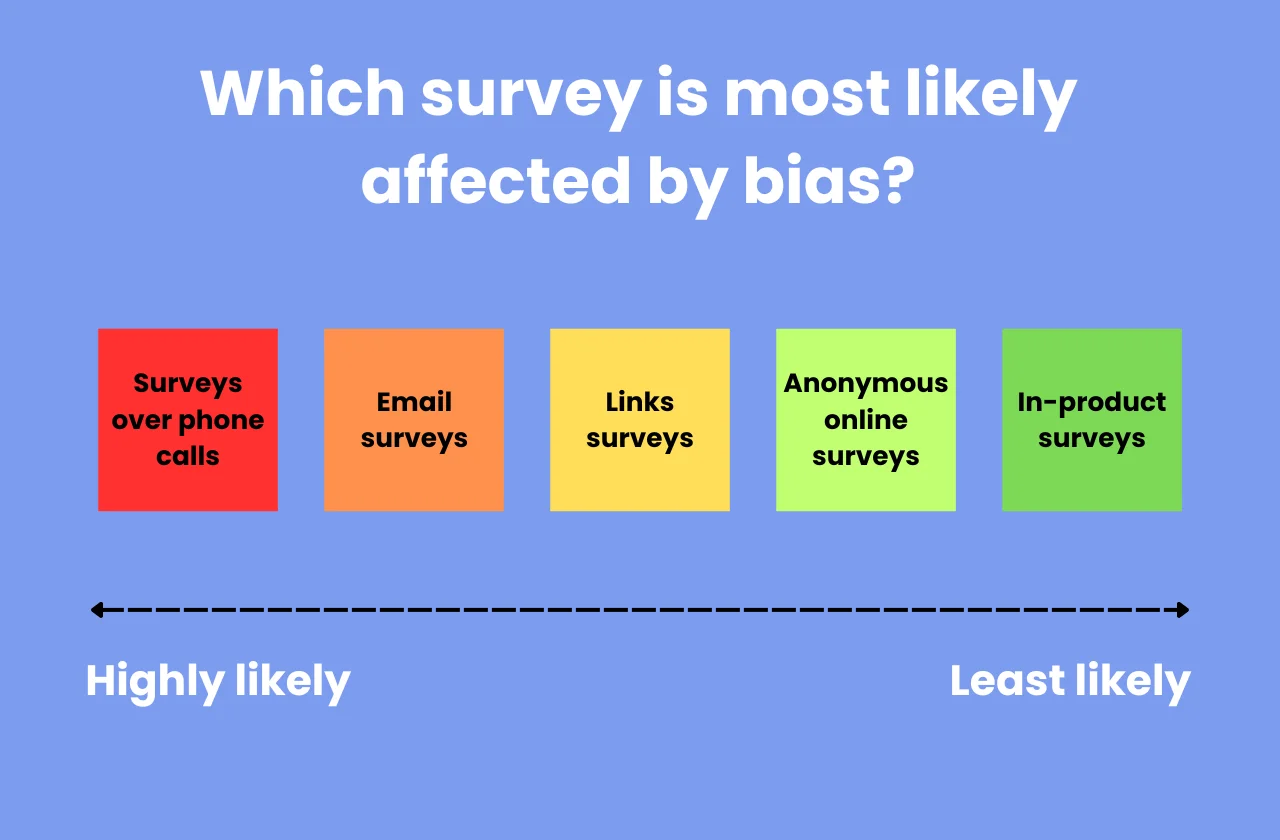
1) Surveys over phone calls
Phone call surveys, though more direct, are not immune to biases and necessitate specific strategies for mitigation.
Recency bias: Users may be more inclined to recall recent experiences, potentially overlooking long-term patterns.
Avoidance: Structure questions to prompt consideration of both recent and overall experiences.
Central-tendency bias: Respondents might opt for middle-of-the-road answers to avoid extreme positions.
Avoidance: Encourage honest opinions by emphasizing the value of diverse perspectives.
Acquiescence bias: Similar to in-product surveys, phone call surveys may face respondents habitually agreeing with statements.
Avoidance: Incorporate diverse question framing to discourage automatic agreement.
Question order bias: The sequence of questions can impact responses, with early questions influencing subsequent answers.
Avoidance: Randomize question order to minimize the impact of question sequence on user responses.
Recall bias: Similar to other survey types, users may struggle to recall details accurately during phone surveys.
Avoidance: Focus on recent and specific interactions, minimizing reliance on memory for responses.
2) Email surveys
Email surveys, widely used for user feedback, are susceptible to specific biases that can impact the accuracy of collected data.
Sampling bias: If the survey is sent only to active users, excluding dormant ones, the feedback might not represent the entire user base.
Avoidance: Use a systematic approach, like sending surveys to a random sample of users, ensuring a diverse representation.
Wording bias: The way questions are phrased can lead respondents towards certain answers. For example, asking, "How satisfied are you with this amazing feature?" implies a positive bias.
Avoidance: Use neutral language and review questions for potential wording biases to maintain objectivity.
Non-Response bias: If only a specific subset of users responds, their opinions may not reflect the sentiments of the silent majority.
Avoidance: Implement follow-up reminders and incentives to encourage a broader range of users to participate.
Recall bias: Users may struggle to accurately recall details, affecting the precision of feedback. For instance, asking about specific interactions from weeks ago may lead to inaccurate responses.
Avoidance: Frame questions in a way that minimizes reliance on memory and encourages respondents to refer to recent experiences.
Current-mood or Emotional-state bias: Responses may be influenced by the user's current emotional state, leading to feedback that does not reflect their typical sentiment.
Avoidance: Consider the timing of survey distribution to avoid peak emotional moments, ensuring a more stable and unbiased response.
3) Links surveys (Distributed over messaging tools or forums)
Surveys distributed via links on messaging tools or forums face unique challenges related to biases.
Non-Response bias: Users active on certain platforms may be more likely to respond, leading to skewed feedback.
Avoidance: Diversify distribution channels to include platforms with a varied user demographic.
Sampling bias: If links are shared within specific communities, the resulting feedback may not be representative of the entire user base.
Avoidance: Use diverse channels to distribute links, ensuring a broad spectrum of users is reached.
Social-desirability bias: Responses may be influenced by the perceived opinions of the user's online community.
Avoidance: Emphasize the importance of individual perspectives and reassure users about the confidentiality of their responses.
Wording bias: The context in which links are shared can influence the user's interpretation of questions.
Avoidance: Ensure clarity in survey instructions and framing to minimize potential biases related to wording.
Recall bias: Similar to other online surveys, users might struggle to recall details accurately.
Avoidance: Design questions that rely less on memory and more on immediate user experiences.
4) Anonymous online surveys
Despite the anonymity provided, online surveys can be affected by various biases that necessitate careful consideration.
Social-desirability bias: Even when anonymous, users may still provide responses they perceive as socially acceptable, impacting the genuineness of feedback.
Avoidance: Emphasize the anonymous nature of the survey and reassure users that honest feedback is valued.
Random-response bias: Respondents may provide answers arbitrarily, especially if they perceive the survey as lengthy or tedious.
Avoidance: Include attention-check questions or captchas to ensure respondents are engaged and providing thoughtful responses.
Prestige bias: Users might provide responses they believe align with a perceived 'prestigious' opinion, affecting the authenticity of feedback.
Avoidance: Frame questions in a way that does not imply a 'right' or 'prestigious' answer, promoting unbiased responses.
Recall bias: Similar to email surveys, online surveys are susceptible to users recalling recent experiences more vividly than older ones.
Avoidance: Structure questions to minimize reliance on memory and encourage users to focus on recent interactions.
Wording bias: The way questions are phrased can lead to biased responses, even in anonymous settings.
Avoidance: Employ neutral language and conduct pilot testing to identify and rectify potential wording biases.
5) In-product surveys
In-product surveys, integrated into the user interface, can be prone to various biases affecting the reliability of gathered data.
Sampling bias: This occurs when the survey sample is not representative of the entire user base. For instance, if the survey pop-up appears only for paying customers, the feedback might not reflect the sentiments of free users.
Avoidance: Implement random sampling to ensure all user segments are equally likely to be surveyed.
Recency bias: Users might primarily recall recent experiences, leading to skewed feedback. For example, a newly introduced feature might receive disproportionately positive or negative reviews due to heightened recency.
Avoidance: Frame questions with a time context, prompting users to consider a broader timeframe in their responses.
Social-desirability bias: Users may provide responses they think align with social norms or expectations, impacting the authenticity of feedback. For instance, users might overstate their usage of a feature to appear more engaged.
Avoidance: Craft questions that focus on specific behaviors rather than general opinions to mitigate the influence of social desirability.
Question order bias: The sequence of questions can influence responses. Placing positive or negative questions first may sway subsequent answers. For instance, asking about satisfaction before functionality could alter perceptions.
Avoidance: Randomize the order of questions to reduce the impact of question sequence on responses.
Acquiescence bias: Some users tend to agree with statements rather than express dissent. This agreement bias can distort feedback by not reflecting genuine opinions.
Avoidance: Mix positively and negatively framed questions to discourage habitual agreement and encourage thoughtful responses.
Having explored the impact of bias on different survey types, let's shift our focus to practical tools that can assist in minimizing survey biases effectively.
Best tools that can help you minimize survey biases
In the pursuit of unbiased data, leveraging technology is crucial. Various tools and platforms are designed to aid researchers and product owners in minimizing survey biases. From survey design to survey analysis, incorporating these tools into your research arsenal enhances the reliability and accuracy of collected data:
1) Blitzllama
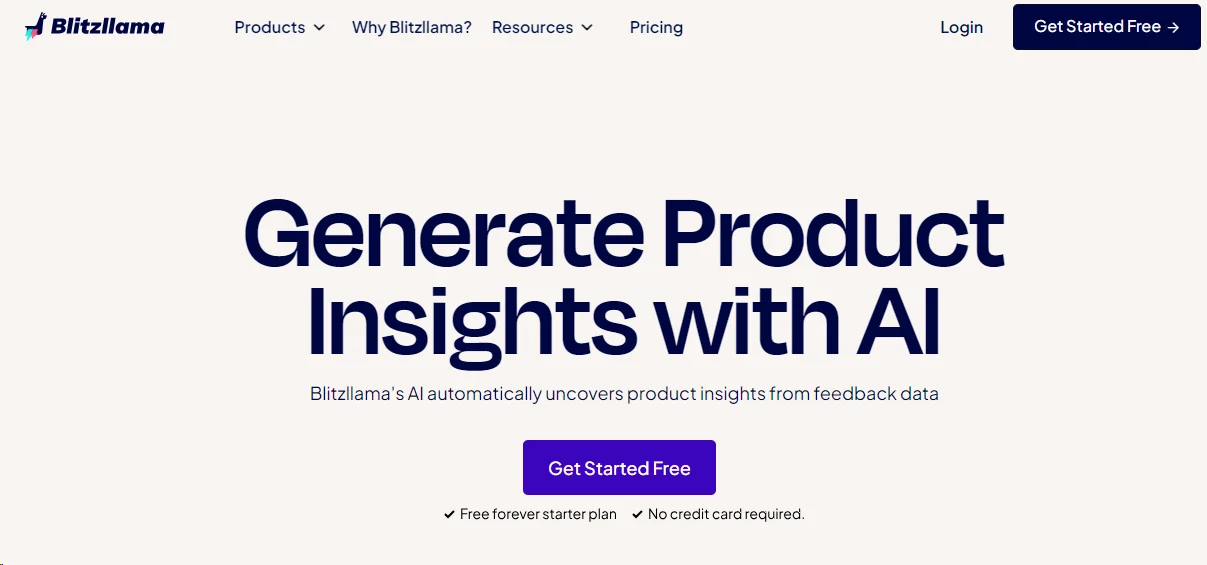
Blitzllama stands out as an exceptional tool for product owners and UX researchers seeking to reduce survey biases. Its AI survey assistant is designed to actively minimize biases by providing real-time feedback and suggestions during survey creation. The assistant identifies potential biased language, helping users craft neutral and inclusive questions. With Blitzllama, the focus is on creating surveys that yield reliable data, ensuring that respondents are not influenced by unintentional biases, ultimately leading to more accurate and actionable insights.
2) Typeform
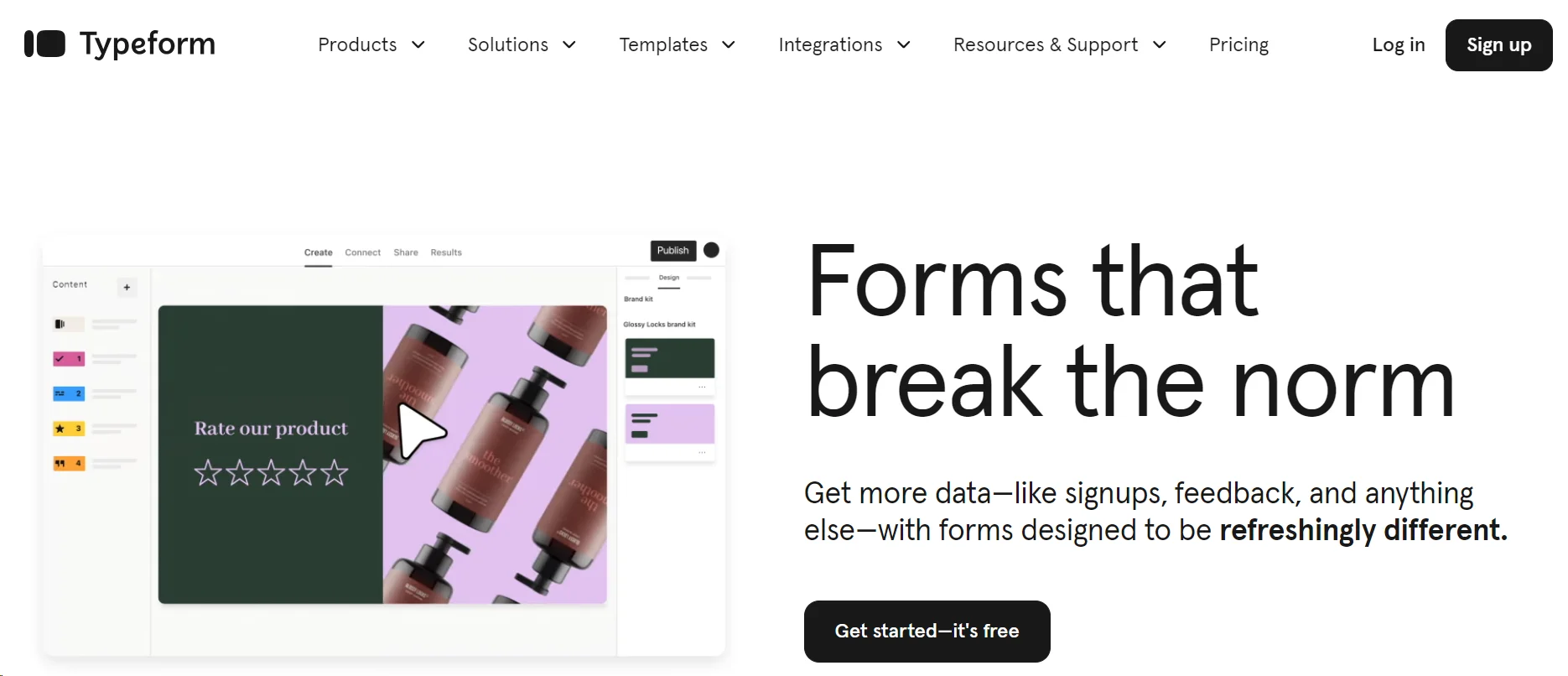
Typeform emerges as a user-friendly tool that empowers product owners and UX researchers to create surveys with minimal biases. Its intuitive interface encourages the formulation of clear and unbiased questions, reducing the likelihood of misinterpretation by respondents. Typeform's dynamic question branching feature guides participants through a personalized survey experience, avoiding unnecessary biases. By offering a straightforward yet sophisticated survey creation process, Typeform enables researchers to collect unbiased data, making it an ideal choice for those prioritizing simplicity and precision.
3) Survey Monkey
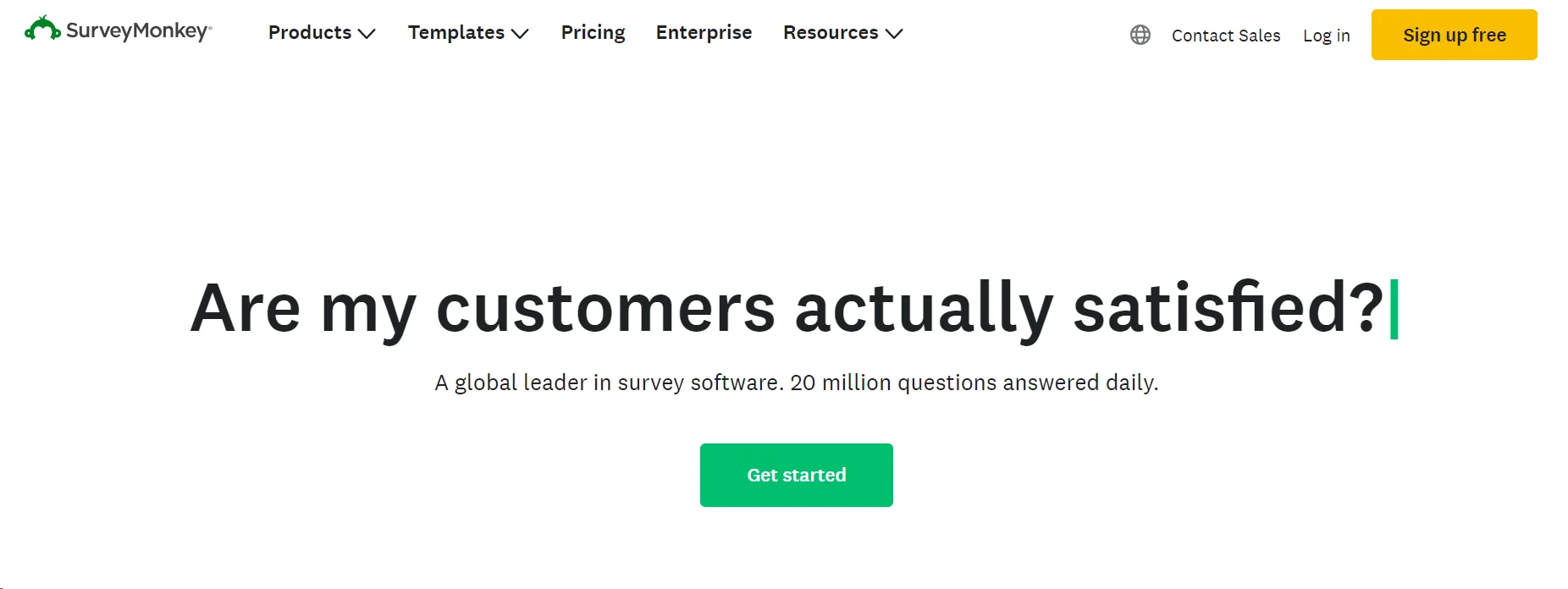
Survey Monkey remains a staple in the toolkit of product owners and UX researchers committed to minimizing biases in their surveys. With its comprehensive set of features, Survey Monkey allows users to implement various question types and survey formats, ensuring diversity in data collection. The platform's expert-reviewed survey templates provide a reliable starting point, reducing the risk of unintentional biases. Additionally, Survey Monkey's analytical tools facilitate the identification of potential biases in responses, empowering researchers to refine their surveys for more accurate and unbiased results. Overall, Survey Monkey offers a user-friendly and versatile solution for those aiming to conduct surveys with minimal biases.
Conclusion
In wrapping up, it's evident that user satisfaction surveys are prone to bias, often influenced by respondent attitudes and experiences.
The risk is higher when using in-app surveys due to their immediacy, potentially capturing emotional responses. While remote surveys offer convenience, they may attract more engaged users, skewing results positively.
Striking a balance between survey methods and ensuring diverse participant representation remains crucial for meaningful insights.
Product owners and UX researchers should remain vigilant, employing a mix of survey types to mitigate bias. Regularly reassessing survey methodologies and refining questions helps maintain accuracy, empowering decision-makers to enhance user experiences effectively.